How to Choose Between Data Lake and Data Warehouse
- dataUology
- Apr 5, 2024
- 3 min read
Updated: Apr 9, 2024

In the fast-paced world of data management, the distinction between Data Lakes and Data Warehouses holds paramount importance. These two concepts represent different approaches to storing, managing, and analyzing data, each offering unique advantages and use cases. In this comprehensive guide, we'll explore the nuances of Data Lakes and Data Warehouses, delve into real-world examples, and discuss potential career paths associated with each.
Navigating the Depths of Raw Data in a Data Lake
A Data Lake serves as a vast reservoir for storing raw, unstructured, and semi-structured data. It is designed to accommodate a wide variety of data types and formats, ranging from text files and images to log data and social media feeds. Unlike traditional databases, Data Lakes adopt a schema-on-read approach, allowing for data to be ingested without predefined structures.
Key Features of Data Lakes
Scalability
Data Lakes are highly scalable and capable of storing petabytes of data from disparate sources. This scalability makes them well-suited for organizations dealing with massive volumes of data generated by IoT devices, social media platforms, and sensor networks.
Flexibility
With a schema-on-read approach, Data Lakes offer unparalleled flexibility in data processing and analysis. Data can be stored in its raw form, and schema can be applied dynamically at the time of analysis, enabling rapid experimentation and exploration.
Cost-Effectiveness
By leveraging cloud storage solutions such as Amazon S3 or Azure Data Lake Storage, organizations can store large volumes of data at a fraction of the cost compared to traditional data warehousing solutions.
Real-World Example
Netflix

The world's leading streaming platform relies heavily on Data Lakes to manage its vast repository of user data, content metadata, and streaming logs. By storing raw data in a Data Lake infrastructure, Netflix can perform advanced analytics, personalized recommendations, and content optimization to enhance the user experience.
Careers in Data Lakes
Data Engineer
Responsible for designing, building, and maintaining Data Lake infrastructure, data pipelines, and ETL processes.
Data Scientist
Utilizes advanced analytics and machine learning techniques to extract insights from raw data stored in Data Lakes.
Big Data Architect
Designs scalable and resilient Data Lake architectures to meet the evolving needs of the organization.
Data Warehouse Insights
A Data Warehouse is a centralized repository optimized for storing structured, organized data. It follows a schema-on-write approach, where data is structured, cleaned, and transformed before being loaded into the warehouse. Data Warehouses are specifically tailored for business intelligence, reporting, and decision support, offering fast and efficient SQL-based querying capabilities.
Features of Data Warehouses
Structured Data Model
Data Warehouses enforce a structured data model, typically in the form of star or snowflake schemas, enabling efficient querying and analysis.
Aggregated Data
Data Warehouses often store aggregated and summarized data, making them ideal for generating reports, dashboards, and key performance indicators (KPIs).
Data Quality and Governance
Due to the structured nature of data, Data Warehouses facilitate data quality management and governance, ensuring data consistency, accuracy, and compliance with regulatory standards.
Real-World Example -- Walmart
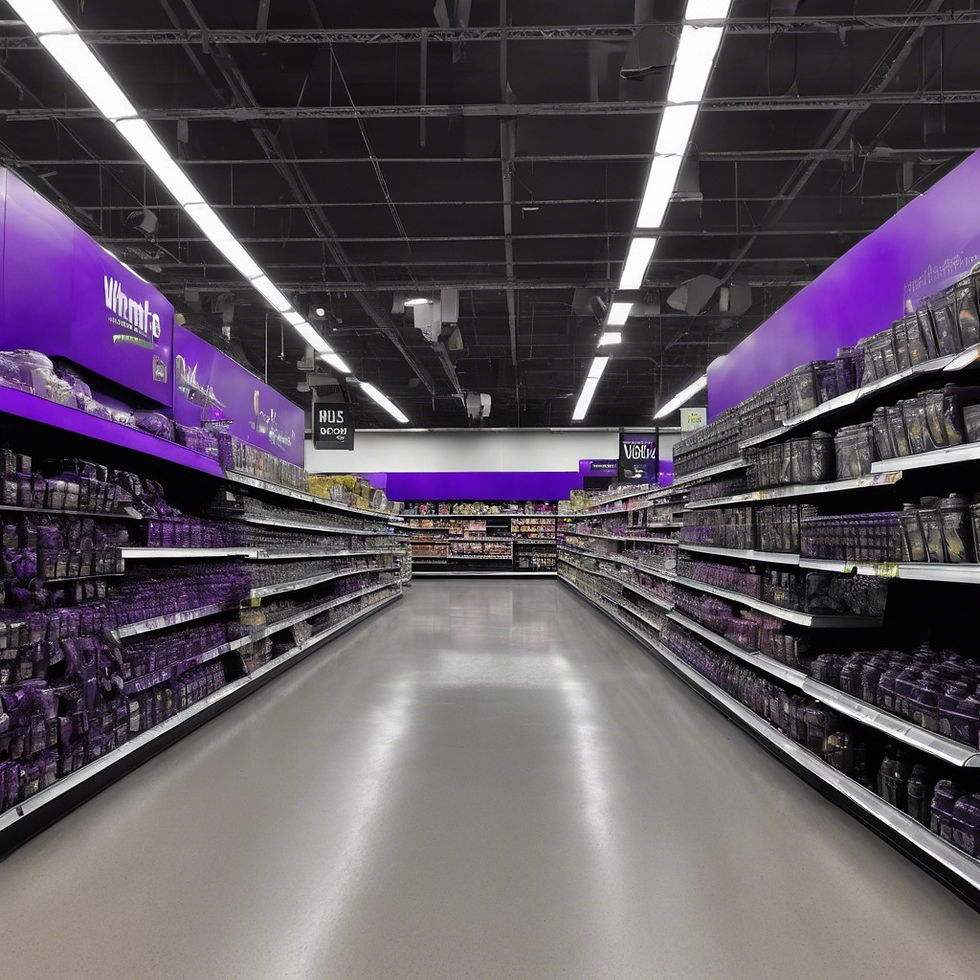
Walmart, one of the world's largest retailers, relies on a Data Warehouse infrastructure to analyze sales data, track inventory levels, and optimize supply chain operations. By consolidating data from multiple sources into a centralized warehouse, Walmart can generate real-time insights, forecast demand, and make data-driven decisions to drive business growth.
Careers in Data Warehouses
Data Warehouse Architect
Designs and implements scalable and efficient data warehouse architectures to support business analytics and reporting requirements.
Business Intelligence Analyst
Develops reports, dashboards, and visualizations to convey actionable insights derived from data warehouse repositories.
Data Warehouse Developer
Builds and maintains ETL processes, data pipelines, and data integration workflows to populate and update data warehouse tables.
Key Differences
Data Structure
Data Lakes store raw, unstructured data, whereas Data Warehouses store structured, organized data suitable for analysis and reporting.
Data Processing
Data Lakes adopt a schema-on-read approach, offering flexibility in data processing, while Data Warehouses follow a schema-on-write approach, enforcing data structure upfront.
Use Cases
Data Lakes are well-suited for exploratory data analysis, data science, and machine learning, whereas Data Warehouses are tailored for business intelligence, reporting, and decision support.
Conclusion
Data Lakes and Data Warehouses represent distinct paradigms in the realm of data management, each offering unique advantages and opportunities for career growth. By understanding the differences between these two concepts and evaluating their respective use cases, organizations can effectively leverage data to drive innovation, enhance decision-making, and gain a competitive edge in today's data-driven world.